Automation in Brain Tumor Diagnosis from MRI Scans - a cutting edge research at PMU center of Artificial Intelligence
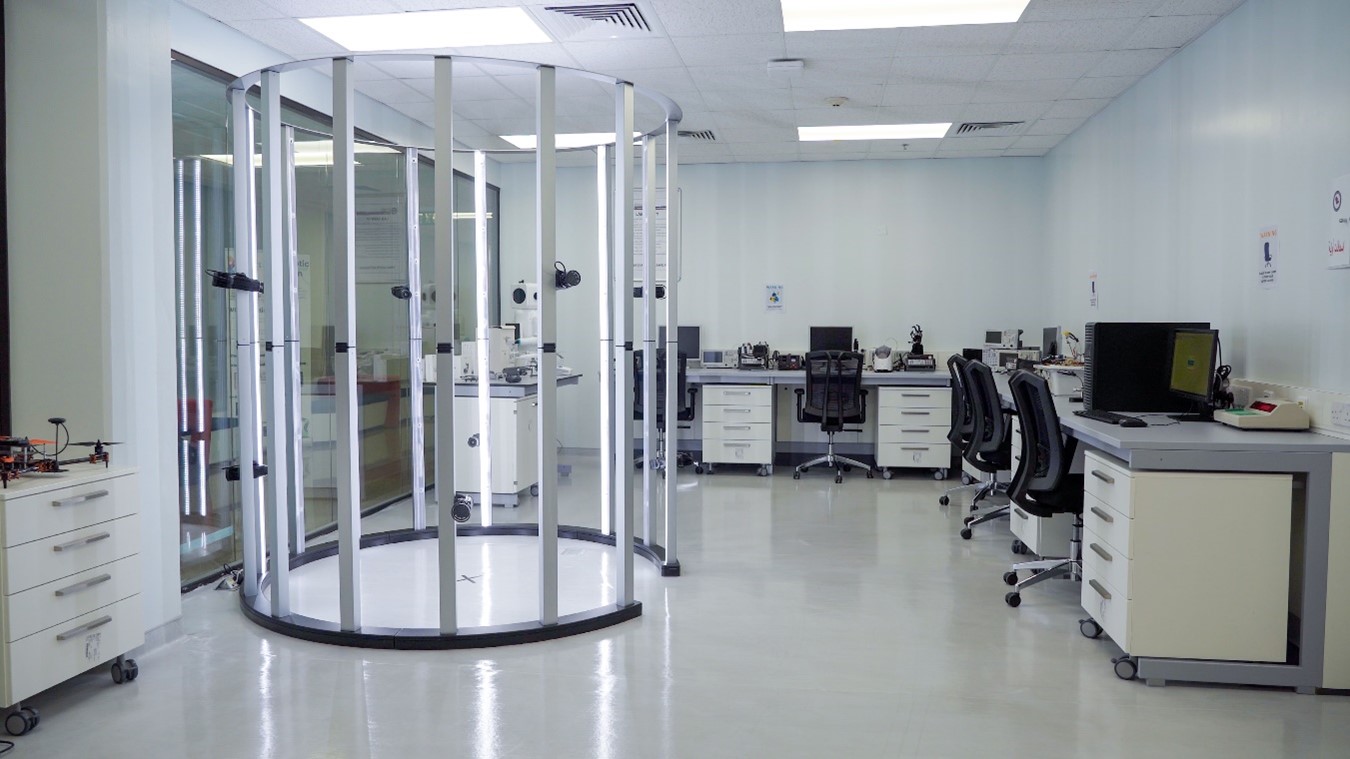
Sponsored by
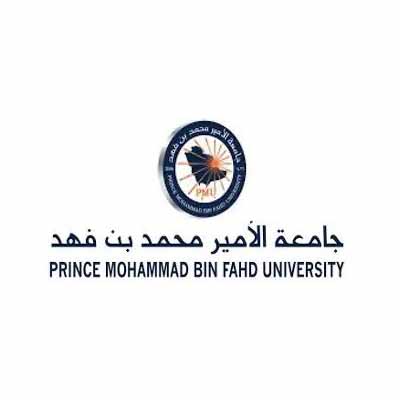
Research into brain tumor is a common research area and common cancer type in Saudi Arabia. Several clinical techniques have been introduced in the past 2 decades for the early identification of this tumor, yet it remains a largely unsolved provocation.
Brain tumors are one of the major causes of death and morbidity around the world. Between 2006 and 2016, Saudi Arabia recorded 1854 and 1293 cases of brain tumors in males and females, respectively. Analyzing brain tumor images taken in a medical context may be incredibly challenging. There is currently a lot of interest in classifying brain tumors using multimodal radiology-based scans. Brain tumors are often diagnosed via magnetic resonance imaging (MRI). MRI is widely used to identify brain tissues based on their size, shape, or location, and can detect a tumor. The manual analysis of brain tumor from MRI scans is a difficult and time-consuming process; therefore, a computerized technique is widely required.
At PMU`s center of Artificial Intelligence an innovative computerized technique based on deep learning and explainable AI for brain tumor diagnosis using MRI scans has been developed the research team led by Professor Attique Khan.
Encoder-decoder-based DeepLabv3+ architecture with Atrous Spatial Pyramid Pooling (ASPP) technique has been developed that detects the brain tumor region from MRI scans.
Two customized models have been proposed named Inverted Residual Bottleneck 96 layers (IRB-96) and Inverted Residual Bottleneck Self-Attention (IRB-Self) that are fused and performed tumor classification such Meningioma, Glioma, and pituitary. An explainable AI technique is also implemented to further check the interpretability of the deep learning models.
The experimental process of the developed deep learning model was established and performed on the publically available MRI dataset, and an average segmentation accuracy of 92.68 % and classification accuracy of 95.42 % were obtained, respectively.
Compared with state-of-the-art techniques, the developed model shows improved level of accuracy.