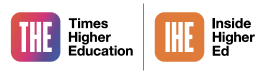
AI and assessment redesign: a four-step process
If GenAI tools have ushered in an era in which institutions can no longer assure the integrity of each individual assessment, the sector must focus on assuring the integrity of awards, write Samuel Doherty and Steven Warburton
You may also like
Popular resources
Risks to the academic integrity of HE assessments have increased significantly with the wider availability and sophistication of generative artificial intelligence (GenAI) tools. Addressing these requires a genuine institutional commitment to staff development, support and time for transformative work.
If GenAI tools have ushered in an era in which institutions can no longer assure the integrity of each individual assessment, the sector must focus on assuring the integrity of awards. There is a growing consensus that to do this, assessments should demonstrate a systemic approach to programme learning outcomes by securing academic integrity at meaningful points across a programme of study rather than at individual course/unit level. But how can we do this sustainably, given the existing pressures on academic and professional staff?
Review and categorise
Step one requires academics to complete a self-review of each assessment task, ie, how likely it is that AI can complete a task. Based on their knowledge and subject matter expertise, staff must consider several risk factors to establish a low/medium/high rating:
Risk factor | Description |
Type | Some task types (for example, a written essay) will have a higher level of risk than others (eg, oral presentation or invigilated exam). |
Context | Tasks based on very specific material, or that involve authentic application to novel real-world situations, are likely to have lower risk than tasks based on more generally available information or basic concepts. |
Conditions | Fully online assessments may involve higher levels of risk than those that involve some in-person component. |
Output | Tasks that involve the creation of an artefact (for example an essay or report) may involve higher levels of risk than tasks that involve a performance component. |
Submission | Tasks that end at submission may have a higher level of risk than those that involve a post-submission discussion or follow-up (for example, a Q&A post presentation). |
Quality of AI output | High-quality AI output is a risk to academic integrity because it may not be recognised as AI output. Staff should test their assessment via a secure GenAI platform and consider the output quality. |
Invigilation | Fully invigilated tasks have a comparatively low risk. |
Map and analyse
Step two involves producing maps detailing the use of assessment tasks (with risk ratings) across programmes. Discipline groups carefully consider and identify tasks that are key to students’ achievement of programme-level learning outcomes. They must make reasoned decisions about whether the potential use of AI compromises the academic integrity of these key programme assessments, or whether there are gaps that need to be filled by potential assessment redesign.
They can then prioritise the critical programme assessments that are identified as at risk for assessment reform initiatives. Prioritisation should include triangulation with other factors such as the number of students enrolled in the programme and its overall strategic importance. Any assessment tasks identified as being of lesser importance to the achievement of programme outcomes may offer opportunities to incorporate the use of AI in learning and assessment to help students engage responsibly and ethically with AI.
- AI resources for higher education professionals
- How can we teach AI literacy skills?
- We need to address the generative AI literacy gap in higher education
Reform assessment
Step three involves the planning of assessment reform initiatives that secure the resources (human and technical) required to implement meaningful assessment reform on a potentially large scale. Learning designers who can help identify and implement appropriate measures to ensure the integrity of key programme assessments must support academic staff at this stage. Approaches will be sensitive to disciplinary norms but are likely to involve a mix of moving assessments to secure testing platforms, for example, online proctoring adding additional components to existing tasks such as a presentation or debate or introducing entirely new task types, such as interactive oral assessments.
Governance
Finally, in step four, given the effort required to undertake large-scale assessment reform, teaching and learning committees should review associated governance mechanisms to ensure an ongoing quality cycle.
Samuel Doherty is the education and innovation coordinator, and Steven Warburton is pro vice-chancellor for education and innovation at the University of Newcastle, Australia.