
Breaking academic barriers: large language models and the future of search
The true potential of generative AI and large language models remains underexplored in academia. These technologies may offer more than just answers. Here’s how the insights they offer could revolutionise academic search and discovery
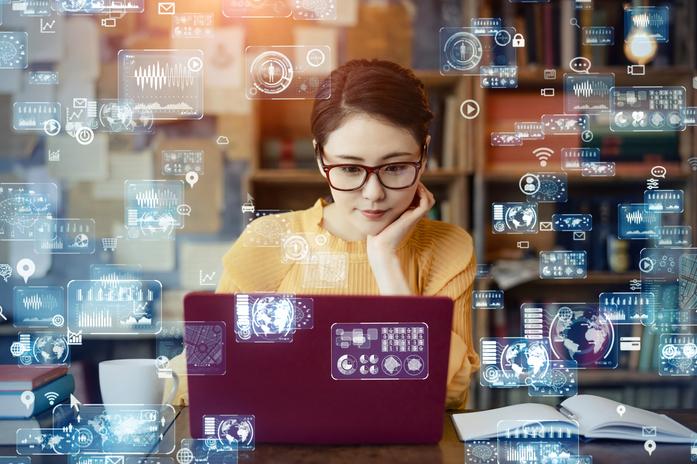
In today’s fast-paced academic landscape, scholars face a rapidly expanding ocean of research articles, so they need search tools capable of more than just fetching documents. The future of search is not just about finding what we seek; it’s about generating deep insights that help us leverage this vast sea of knowledge.
At Elsevier, we are working with the academic community to co-create search engines to meet the scholarly needs of our community.
- Collection: AI transformers like ChatGPT are here, so what next?
- Streamline your research using academic search engines
- Three ways to refresh your academic writing
We’re entering an era where large language models (LLMs) have the potential to revolutionise the way we search and discover information. When paired with trusted academic databases, these models promise to become more than just text generators; they transform into advanced knowledge engines. They can sift through massive databases, draw connections between disparate fields and even suggest new avenues of research. In this way, they don’t just find information but also help us to understand its broader context and significance, unlocking the full potential of academic resources.
Take Microsoft’s Bing ChatGPT as an example. It demonstrates an impressive application of LLMs in search, but this only scratches the surface of what’s possible. Generating summaries or answers based on web results is helpful. Still, the true value of LLMs lies not in their ability to find information but rather in their capacity to reframe it in a way that helps us to comprehend its significance.
Picture an academic philosopher deeply immersed in research on ethics and values. Through the power of LLMs, a platform could provide thought-provoking perspectives and suggest how research in the field of, say, synthetic biology applies to their work. A biochemist could find their research enriched by insights from quantum computing. The French Revolution could be illuminated to historians through the lens of game theory. This isn’t sci-fi or fantasy; it’s what domainless LLMs can bring to academic enquiry today.
Practical ways to use large language models for reflection
The stakes couldn’t be higher. As knowledge expands, researchers must narrow their focus and master their domains’ growing specialised language. We are locking ourselves into ever-narrowing disciplinary vocabularies and worldviews, as the necessity of remaining experts in our fields truncates our capacity for broad-mindedness. Consider economics’ heavy reliance on mathematical models and statistical analyses, which can create a barrier for those without a strong background in either subject. This leaves the rest of us on the outside looking in, unable to access knowledge that otherwise could help us to create new insights or make informed decisions.
The implications of increasing specialisation are worrying because some of the most remarkable breakthroughs in human history have come from the confluence of disparate fields. One of my favourite examples is the Massachusetts Institute of Technology’s Building 20, where researchers, scientists and even janitors roamed each other’s labs, sharing ideas and inspiring bold new concepts. Interdisciplinary collaboration led to groundbreaking innovations in high-speed photography, microwave physics and even the creation of the Bose Corporation. This creative melting pot, which stood from 1943 to 1998, gave birth to the first video game and pioneering research on Chomskyan linguistics.
With LLMs, we have the potential to provide a Building 20 for everyone.
One practical step academics and researchers can take now is to start experimenting with LLMs for self-reflective exploration. These models can serve as an intellectual sounding board, capable of generating Socratic-style questions. For instance, if you’re a physicist studying particle behaviour, a well-designed query to an LLM could prompt questions such as: “How might my understanding of particles contribute to advances in medical imaging?” or “Could my research have implications for environmental science?” Such questions not only foster interdisciplinary thinking but also offer a form of self-study and way to broaden academic horizons.
Limitations of large language models for academic enquiry
It’s crucial to note that while LLMs offer much, they are not a cure-all. LLMs, by default, are designed to generate convincing-sounding text, not to produce factual statements often referred to as “hallucinations”. Second, these models are constrained by the data they were trained on, meaning that their “knowledge” can quickly become outdated, especially in fast-evolving disciplines – a critical point, given that the cornerstone of scientific research is accuracy and timeliness.
That said, even these limitations can be harnessed creatively. For example, hallucinations could be instrumental in sparking new connections or theories between disciplines when used judiciously, turning a potential weakness into a novel form of enquiry.
LLMs represent the first significant advancement in search and discovery in decades. Best of all, this is only the beginning, and we are starting to see fine-tuned optimisations already.
For example, we recently released an early version of Scopus AI for researcher testing. This next-generation tool combines generative AI with Scopus’ content and data to help researchers get deeper insights faster and support collaboration.
LLMs will enable us to go beyond mere recall, fostering contextual understanding and innovative discovery. Our goal should not just be to build better search engines but to craft more intelligent, intuitive tools that enrich the pursuit of knowledge itself.
Adrian Raudaschl is a senior product manager at Elsevier.
If you would like advice and insight from academics and university staff delivered direct to your inbox each week, sign up for the Campus newsletter.