For responding too late to warning emails, my son was declared to have ‘failed to abide by the attendance policy of the university’
As an academic, I have learned much from watching how my own children have fared at university. The migraine suffered by one of them ahead of an assignment deadline of midnight on Sunday convinced me to set all my subsequent deadlines for 5pm on working days. Seeing another trying to revise from just 24 slides prompted me to build cumulative packs of slides for easy access. Noting the loneliness of en suite private accommodation, I consistently spread the message that accommodation design matters.
The effect of automated attendance monitoring systems came into my focus when, with two teaching weeks left and exams looming, my son was withdrawn for poor attendance from the first year of his maths course.
Newly installed at my son’s UK university, the student attendance monitoring (SAM) system was described as a student-friendly tool that would, in the words of the pro vice-chancellor, “allow us to see if someone is struggling and…to offer support as soon as possible”. Such systems, of course, also neatly deal with universities’ legal requirements to monitor the attendance of international students, and help to identify (in a crude correlation of attendance and achievement) students who might fail and, thereby, lower scores in the teaching excellence framework.
Such systems typically send auto-generated emails to students warning them of the consequences of non-attendance and suggest ways to get in touch with someone if they need help. My son was sent four such emails. The first was in early December. He didn’t notice the second, in late February, and he replied to the third, sent in mid-March, too late: three days before the fourth and final warning was sent. He was thereby declared to have “failed to abide by the attendance policy of the university, despite being contacted repeatedly”.
What had gone wrong was that, first, he had not understood what was expected of him. He had failed to compute that when his coursebook said that he was “being asked to scan your student ID card at entry points into most lecture rooms”, it meant that he was being required to do so (he hadn’t even had a student card to scan during the first semester). When he appealed against his withdrawal, noting that he hadn’t had much time to respond between reading email three and receiving email four, the adjudicator wrote that he had a “duty to read email”. That was news to him, too.
Second, you have to question whether a system intended to “offer support as soon as possible” should wait until six or more weeks into the teaching term before emailing a student who has, according to scanned-in data, been to no classes at all. By this point, habits have been formed. Even if the student reacts by then attending a few classes, engagement with the course is already shaky. Such cases surely require earlier and probably more personalised intervention – involving, perhaps, a visit from a second- or third-year student to see what can be done.
Third, my son was studying during the early adopter phase of a new system. SAM systems change the behaviours of academics. My son had initially completed a couple of weekly worksheets, but not one of the five academics who were teaching him got in touch after his hand-ins dried up. These were, in my son’s words, “great lecturers”, but they probably felt that tracking their students was no longer their responsibility, and they were probably unaware that the SAM system was not taking care of these things, either. I say this because I reacted in exactly the same way to the implementation of a SAM system at my own institution.
My son and I move on. He has no regrets, and I want no replays. He is figuring out what to do when a degree is no longer an option, and I am trying to unpick what strategy would help guys like him “hack education”, as he puts it.
My conclusions so far are that universities should be required to ensure that students fully understand requirements around reading emails and SAM systems. Potentially life-changing emails should be sent with “have read” receipts, and should be followed up with further warnings, including physical letters, if they are not read. And staff should be clear about what their institutions’ SAM systems are doing and be able to influence the behaviours of such systems to support students in developing effective learning habits.
It may be that my son was always going to do what he did. But it is at least conceivable that things might have ended differently if the SAM system had been better embedded. Successfully implementing a new technology takes time; the university admitted that when it warned at the outset that “there may be teething problems…so please be patient”. Why, then, did it come down so harshly on someone who fell through the safety net that it was supposed to be providing?
My son was at least lucky that he had me to talk to and also had the support of friends as his university adventure came to an abrupt halt. The ending could have been much darker had he been alone with a migraine in a luxury en suite room.
Janet Read is professor of child-computer interaction at the University of Central Lancashire.
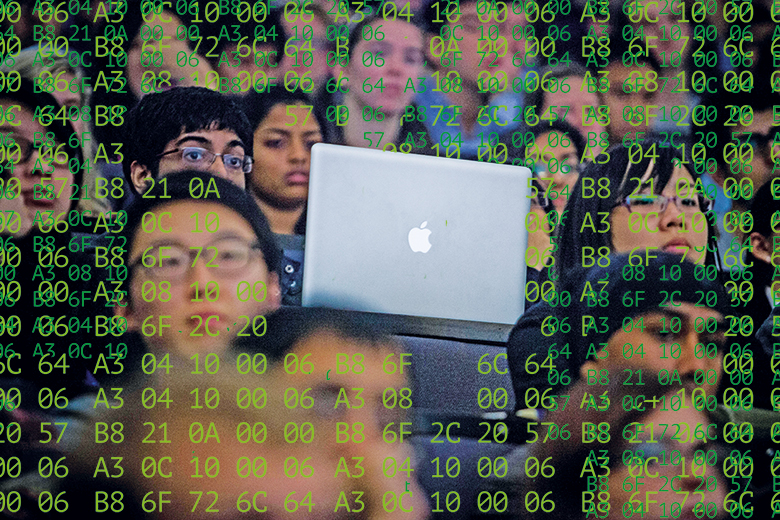
Learning analytics can help students identify their most suitable time of day to learn, and which methods are most effective for them. And it can allow lecturers to insist on more engagement from students
We are in the age of data. Just look around: everyone has a smartphone or an activity tracker analysing their heartbeat, counting the steps they take, and monitoring how many hours they sleep. At the end of their day, owners of these devices can see exactly how they spent their time. What if we could do that for student engagement?
With learning analytics, it is possible to see how students learn and which resources they choose. This has huge potential for e-learning resources in higher education. We can now visualise, click by click, how individual users interact with resources. We can not only see that they got a 55 per cent, but we can pinpoint areas where they went wrong and, from that, work backwards to find out why. We can analyse student interaction with simulated environments, virtual patients (for medics and vets), videos, e-books and quiz-style applications.
Each student’s unique “learner record store” allows them to see how they have spent their time learning and how well they have performed, including relative to current and previous cohorts. Over time, students can identify their most suitable time of day to learn, and which methods are most effective for them. Similarly, students’ working habits can be reviewed by staff to identify those struggling and to suggest remedies, minimising the chances of their dropping out.
But the benefits of learning analytics are not all one-way. They also offer academics a significant boon. Many universities currently use student evaluations when assessing a teaching academic’s promotion application, but anecdotal evidence suggests that the results of such exercises can be biased by a lack of student engagement with modules that are challenging and associated with a higher failure rate.
Using learner analytics, we can identify users who engage with material and those who do not. In my view, it makes perfect sense to “red flag” a student’s feedback if they have not met a predetermined threshold of engagement. It would allow lecturers (and their assessors) to view feedback from those students differently – or even discount it altogether. As well as offering protection for academics who have more challenging modules to teach, this would also allow content creators a chance to use interaction feedback to refine their resources, combating information overload.
Educational theory reminds us that retention of information and levels of understanding are increased by active learning. Many academics have tried to adapt their sessions to include active learning, such as introducing quizzes and discussions into lectures, or providing e-learning resources in various formats, including games. Learning analytics will allow them to much more accurately assess the effectiveness of these interventions.
Furthermore, learning analytics can help staff tackle the resistance to active learning that students typically show. This resistance arises from the fact that active learning is much more challenging than traditional, passive instruction and, as a result, requires more effort. Academics who introduce such innovations into the curriculum can therefore suffer in student assessments and may retreat into exam-focused teaching by rote. The risk is that this kind of approach leaves students underprepared for life after university.
I am not suggesting that we assess students on the basis of their engagement rather than their exam or dissertation scores. But the introduction of learning analytics could provide insight into whether increased engagement with course material correlates with attainment and, if so, galvanise lecturers’ efforts to insist on engagement without worrying how that might affect their scores in student evaluations.
Of course, students will in all likelihood oppose the introduction of learning analytics, condemning it as some kind of sinister, Big Brother imposition. But the strongest resistance is likely to come from the least engaged, who fear being rumbled. Those students need to ask themselves whether they are attending university to enhance their cognitive ability or simply in an attempt to prolong their time as schoolchildren before actively having to challenge themselves.
Simon Patchett is a final-year veterinary student at the University of Nottingham. He recently intercalated a postgraduate certificate in veterinary education and became an associate fellow of the Higher Education Academy.
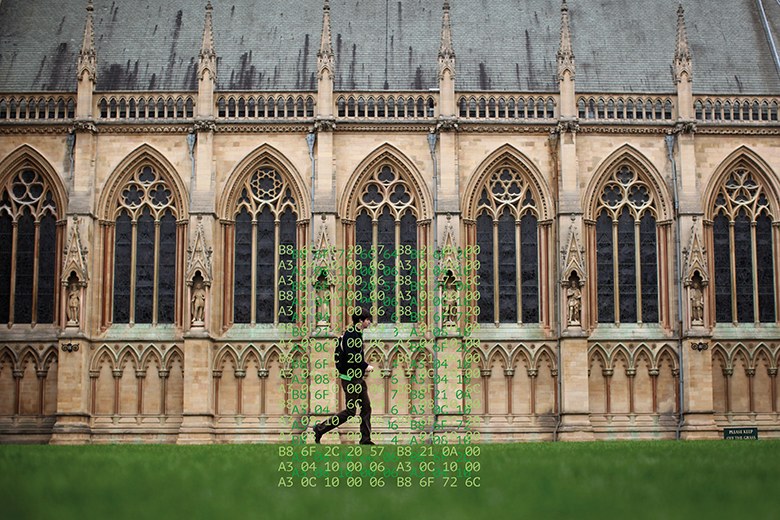
It may be the case that the online shopping metaphor that underpins this approach to student learning is incompatible with the aims of higher education conceived as an ethical project
In a world of escalating accountability and competition, higher education managers are increasingly turning to automated learning analytics systems to guide both their own and their students’ decision-making.
Learning analytics now provide students with advice about which courses to take, and where and how they need to improve. In the flipped classroom model, they are even taking on the role of tutor, making the work of the academic more to do with the tracking and monitoring of student progress than imparting the accumulated wisdom of years of immersion in intellectual endeavour.
All this stems from the recent transformation of the landscape in which universities operate. The demographics of the student body have changed profoundly with the massification and commoditisation of higher education. At the same time, the growth of student numbers has been accompanied by reduced opportunities for personal guidance and tuition as staff-to-student ratios deteriorate.
Global comparison, competition and enhanced accountability have led to increased sensitivity to the risks of student failure and dropout. The UK’s teaching excellence framework is the latest manifestation of increased scrutiny of student satisfaction, completion rates and destinations data. The temptation to pore over the data produced as a by-product of online learning is thus readily understandable.
Increasingly, students and staff who log on to their institutional “learning management systems” are presented with “performance” data. For staff, these include “retention centres” that identify students at risk of failure, while students are presented with “dashboards” that display measures of progress and suggest learning activities or directions. These provide managers with new and varied quantitative metrics on retention and engagement hours that seem readily transformable into league tables or performance targets, and on which decisions at the micro, meso and macro levels might be made.
But unlike carefully designed experimental studies, learning analytics are parasitic, relying on incidental data generated as students pass through the university system – data that are then analysed through the application of algorithms designed in largely non-theoretical ways. In fact, these systems are mostly derived from the field of business intelligence. The recommendation algorithms on shopping sites such as Amazon (“customers who bought this also bought…”) are being implemented to suggest alternative readings or learning activities to students and their teachers.
Enthusiasts claim that these approaches herald a brave new world of personalised learning, in which exactly the right advice and prompts are provided at exactly the right time. But empirical research into the effectiveness of such systems remains ambiguous. Part of the reason might be variations within the student cohorts studies, and the different contexts across disciplines, programmes, year levels and modules. But it may also be the case that the online shopping metaphor that underpins this approach to learning is incompatible with the aims of higher education conceived as an ethical project.
Recent research in which we have been involved has looked at this. We studied different data types from an online master’s-level module at the University of Stirling for practising schoolteachers and compared them with qualitative indicators of learning. These included submissions to the module, which took the form of blog posts and online comments written by students, short essays and contributions to discussion forums about other students’ work.
We found that the quantitative data did indeed exhibit patterns that might be recognised through machine learning, and so be amenable to analysis and metric-generation in learning analytics systems. However, we also found highly complex behaviours and correlations. It was clear that for some students, high levels of online engagement were no indicator of successful learning outcomes, while for others, significant learning was possible despite levels of online engagement that would have most likely triggered warnings of failure in automated systems.
Our experiments with do-it-yourself learning analytics highlight the dangers of any system that drives student behaviour towards a statistically measurable norm that is unlikely to represent the best learning path for every individual. For example, recommender systems of the type used in business intelligence software may not only be difficult to adapt to learning environments but could even be counterproductive if the wrong advice is given to the wrong student.
We do not wish to suggest that the kinds of data that drive learning analytics are never useful. However, it seems clear that universities, departments, course conveners and individual academics need to take a cautious, critical approach, exploring in their own contexts the limits of what can be ascertained from click-based learning data.
Anna Wilson is a research fellow in the division of sociology at Abertay University and adjunct associate professor in the Research School of Physics and Engineering at the Australian National University. Cate Watson is professor of education at the University of Stirling.
POSTSCRIPT:
Print headline: Measured steps
Register to continue
Why register?
- Registration is free and only takes a moment
- Once registered, you can read 3 articles a month
- Sign up for our newsletter
Subscribe
Or subscribe for unlimited access to:
- Unlimited access to news, views, insights & reviews
- Digital editions
- Digital access to THE’s university and college rankings analysis
Already registered or a current subscriber? Login