
How to handle data ethically and effectively as a social scientist
New data sources are generating vast amounts of information for researchers. But social scientists and management researchers should put robust practices in place to remain ethical
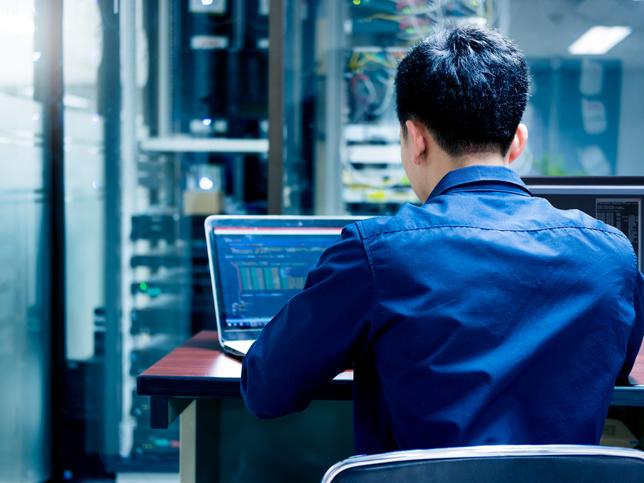
The rapid rise of new technologies in this century has meant that not only are we increasingly seeing new ways to collect data, but we are also seeing new things to collect data on.
In 2008, the launch of Glassdoor.com gave management researchers and academics data that we’d never seen before. Previously, anonymous reviews and salary data from employees were hard to obtain. As scholars were given the insight to ask new questions or approach existing ones from a fresh angle, organisational research boomed.
- Equipping students with data skills to navigate an AI-driven world
- Finding a community and career through data skills
- Enhancing research integrity and impact in APAC using data
More recently, three major trends have created new opportunities for harnessing new data sources. First, we can now gather valuable data in new ways, using cutting-edge technologies such as wearable health monitors and advanced imaging techniques. Second, the cost of field experiments has fallen and firms and other organisations now often run their own experiments, creating fruitful partnerships between researchers and industry.
Third, social media, artificial intelligence, blockchain and sensor-enabled devices are generating vast amounts of “big data” through their digital footprints.
However, as opportunities to use new data arise faster than best practices can be established, there are a number of challenges that come with this. To embrace the full potential of this new data while remaining ethical, we need robust practices and recommendations in place for management researchers and other social scientists.
That is why, in a recent editorial I wrote with my fellow editors at the Academy of Management Journal, we outlined two key recommendations for management academics using these new data sources for research purposes.
Take data context seriously
When using new data sources in research, provide detailed context about the data’s origin, compilation and purpose. Unlike more established databases, new datasets require thorough background information to enable accurate interpretation. For example, researchers using video data to study leadership emotions must justify how the emotions in recorded speeches represent broader leadership styles.
Similarly, when employing text-mining techniques on corporate filings, it’s crucial to explain who compiled the reports, the motivations behind them and any legal requirements that might influence what is included and what is left out. Conducting targeted interviews or providing contextual information that tells the story of how the data came about can significantly enhance the credibility and relevance of the data. It also instils confidence in the reader that the data accurately represent what the authors claim it does.
Contextual clarity also ensures the data’s representativeness and accuracy in reflecting the broader phenomenon being studied. For example, data from public forums may not represent the full breadth of societal discourse on a particular topic. Similarly, when using longitudinal data, be aware that what is and is not included in the dataset might have shifted over time – due to regulatory changes, for example.
Management researchers using audio or video data must consider how the recording process, such as in board meetings, might influence behaviours or decisions. Due to the Hawthorne effect, people can change their behaviour when they are aware of being recorded. When we can acknowledge these limitations – and mitigate them as much as possible – we can help to align theoretical arguments with empirical reality.
This is particularly important when using machine-learning approaches to coding and other forms of data processing and interpretation, where simplifying assumptions and disregarding contextual factors such as economic shocks or decision-maker constraints and biases can skew results. Providing historical, institutional or geographical context, along with sample data, can offer a clearer understanding of the data’s relevance and integrity.
Focus on data transparency and ethical considerations
Transparency and ethical concerns are particularly crucial when adopting new data sources. How data were obtained, processed, analysed and stored must be made clear. Management researchers should follow best practices such as pre-registration of study design, ethical review and making anonymised data available for peer review.
Data usage must comply with data agreements, ownership rights and consent procedures. When using social media or AI-generated data, authors should address potential risks such as data manipulation or fake accounts. Make detailed disclosures available, possibly through online appendices, to maintain transparency and reproducibility.
Additionally, transparency in data handling is critical. For machine-learning methodologies, researchers must show how patterns might vary with different approaches or training datasets to avoid biases. Current Large Language Models, for example, may perform well in specific cultural contexts but poorly in others, highlighting the need to be aware of representational biases.
Field experimenters must balance scientific rigour with the potential harm to participants. For instance, designing experimental conditions that carefully control the main factor of interest can create adverse effects for participants or undermine experimental realism. Researchers should clearly define stopping rules for experiments to mitigate potential harm, ensuring ethical considerations are integrated with scientific objectives.
Mobilising new data sources presents challenges. But, at the same time, it provides exciting opportunities for advancing management and organisational research. The process often requires new analytical techniques and, due to the increased complexity and additional scrutiny this might invite, can deter some authors. However, using these new data sources will allow us to conduct further innovative and ground-breaking research – so we must learn how to deploy it ethically and effectively.
Anne ter Wal is professor of Technology and Innovation Management at Imperial College Business School and is currently serving as an associate editor at the Academy of Management Journal.
If you would like advice and insight from academics and university staff delivered direct to your inbox each week, sign up for the Campus newsletter.