
Reshaping assessment to outsmart AI
Adapting common assessment types can not only deter the overuse of AI – and avoid administration overload – but support student learning in a more authentic and engaging manner
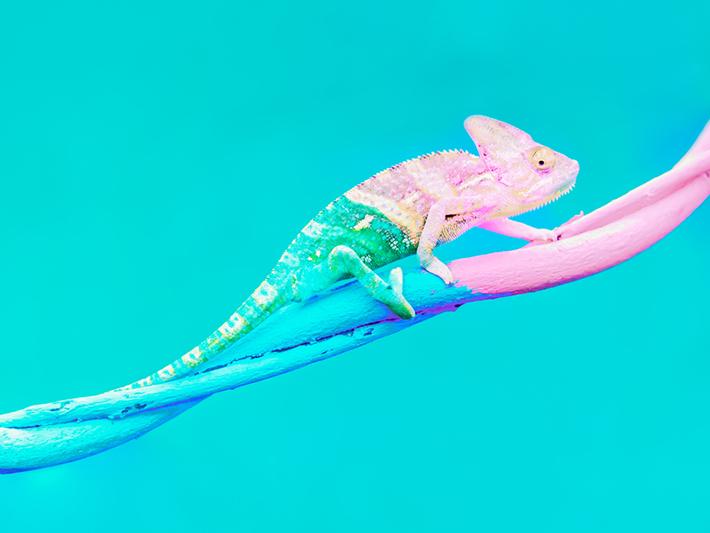
You may also like
Popular resources
When even tools like Grammarly can now substantially alter text – potentially obscuring the academic voice rather than simply supporting the mechanics of writing – it’s clear that AI has changed the rules of engagement around assessment. The arrival of generative AI has seen universities grappling with the need to revise assessment strategies to minimise the risk of students submitting AI-generated responses. However, modifying assessments is not straightforward, often requiring lengthy and complex revalidation processes to align with module and course specifications.
On top of that, academic staff are frequently tasked with investigating suspected breaches of academic integrity linked to the use of GenAI tools such as ChatGPT. Ironically, the time spent on such investigations can exceed the time it might take a student to generate the content. While some institutions offer guidance to help academics identify probable AI-generated content, detection remains unreliable, with high error rates and frequent false positives – even when using AI-detection software. This added layer of complexity has increased administrative workload and delays, and caused widespread frustration and pressure across the sector.
- Resource collection: AI transformers like ChatGPT are here, so what next?
- Spotlight guide: The evolution of authentic assessment
- Campus podcast: The pros and cons of AI in higher education
So, rather than looking at reactive responses at the point of submission, we have been exploring proactive assessment practices at our respective institutions. Our approach reframes established assessment types in ways that are both familiar to students and compliant with documentation and regulatory frameworks. In doing so, we aim to preserve academic integrity while reducing administrative strain. These adapted assessment strategies not only deter the overuse of AI but support student learning in a more authentic and engaging manner.
The table below suggests modifications to common assignment types such as essays, literature reviews and reports that mitigate the risks of students using AI. These adjustments can be easily integrated into your assessment and allow for responsible use of AI, if desired, while still ensuring the task meets the intended learning outcomes of the module.
How to use the table
- Select the assessment type that is attached to your module.
- Review the suggested modifications. Our suggestions do not typically require going through formal module amendment processes.
Assessment type | AI-aware modifications |
Essays | Set critical essay tasks involving an ethical dilemma that requires students to take a position. This position is preferably personal and related to student experience. |
Literature reviews | Allow students to use AI tools such as Research Rabbit to search for relevant literature and explore connections between works or visualise publication timelines. Students could include screenshots of their searches and explain how the argument has developed over time or highlight key similarities between papers. |
Reports | Force strategic choices. Specify which type and year sources must come from (for example: “Use no more than two sources from 2020 and one section MUST include a graph or infographic”). |
Research proposals | Add a metacognitive layer to the task of writing a research proposal. For example, ask students to include a section where they justify their approach and reflect on how their proposal has developed over time. Include discussions and a verbal justification of methodology. |
Dissertations | Require a positionality statement – and get each student to articulate why their positionality is relevant to their work. Include progress check-ins and a short viva to verify the authenticity of the work. |
Short-answer questions | Make short-answer questions application-based. Require examples from students’ personal experience or class discussions. Use varied question sets for different students. |
Lesson plans or curriculum design | Allow students to use an AI tool such as Magic School, Curipod or TeacherMatic to create lesson plans or design curricula. Require critical reflection on potential biases, creativity, alignment with intended learning outcomes, and accessibility. Students must integrate specific contextual factors (such as school demographics or learning needs) and present a brief in-class justification. |
Annotated bibliographies | Invite students to use tools such as Notebook LM to create mind maps, summaries or podcasts. Encourage presentation of sources as visual artefacts – such as infographics or concept maps. |
Blog posts | Ask students to create blog posts based on their personal experience. Encourage reflective writing and digital content creation that demonstrates their developing critical thinking and communication skills. Require inclusion of reflections or multimedia elements (for example, voice recordings or images). |
Academic posters | Introduce poster presentation sessions where students discuss their work in a way that emulates academic conferences. Set in-class presentations where students answer questions about their work. Use real-world data or primary research where possible. |
Annotated photograph/artefact | Provide students with a specific stimulus image or artefact (this could even be a graph, if relevant). Ask students to respond to it by drawing links and connections among this and other aspects of the module. For example, use a graph where they can assess the bias provided by the researcher or discuss problems with the data set. Alternatively, start with a photograph of a classroom and ask students to identify the relevant aspects of an educational approach that may be evident, connecting these to the literature. Students must provide an original photograph or artefact with a written or spoken personal narrative in class. |
In addition to being more resilient to AI misuse, these assessments require students to actively engage with the process of knowledge production. This offers greater opportunities for deep learning and metacognitive development. When the assignment process is more transparent, students are required to be actively aware of how they construct understanding as well as absorb the information. This also opens the way for increasingly accessible education rather than maintaining an unspoken, hidden curriculum. Such approaches go part way to offering more equitable access to learning, as well as richer educational experiences for all.
Rebecca Mace is a senior lecturer at the University of Worcester. Viktoria Magne is an associate professor; Sarah Hooper is a lecturer; and Sharon Vince is a senior lecturer, all at the University of West London.
If you would like advice and insight from academics and university staff delivered direct to your inbox each week, sign up for the Campus newsletter.
Comments (0)
or in order to add a comment